※ INTRODUCTION:
As a crucial post-translational modification, protein phosphorylation is involved in regulating a broad spectrum of biological processes in prokaryotes.
Here, we report an updated server named group-based prediction system for prokaryotic phosphorylation language model (GPS-pPLM), for the prediction of prokaryotic phosphorylation sites. For predictor training, we integrated the transformer-based protein language model and Deep Neural Network, and used 44,839 non-redundant p-sites in 16,041 proteins of 95 prokaryotes. We first pre-trained two general models, which were subsequently fine-tuned to obtained single residue sites model and species-specific model. We obtained 6 residue type site predictors and 134 species-specific predictors. For user, protein sequences in FASTA format or UniProt accession numbers can be input, and the predicted results are displayed in tabular form. We also utilize 22 public resources to annotate phosphorylation sites, including experimental evidence, physical interactions, 3D structures, and disorder tendencies.
For the help of GPS-pPLM and the tutorial, please refer to the USER GUIDE page.
For the source code of GPS-pPLM, please visit the GitHub page.
▼ Example
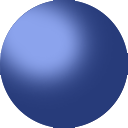